The terms “artificial intelligence” and “machine learning” are highly overused; however, they are loaded with potential for enterprises pursuing the full value of digital transformation. To cut through the hype, it is important to understand their meaning and use in the world of data and analytics.
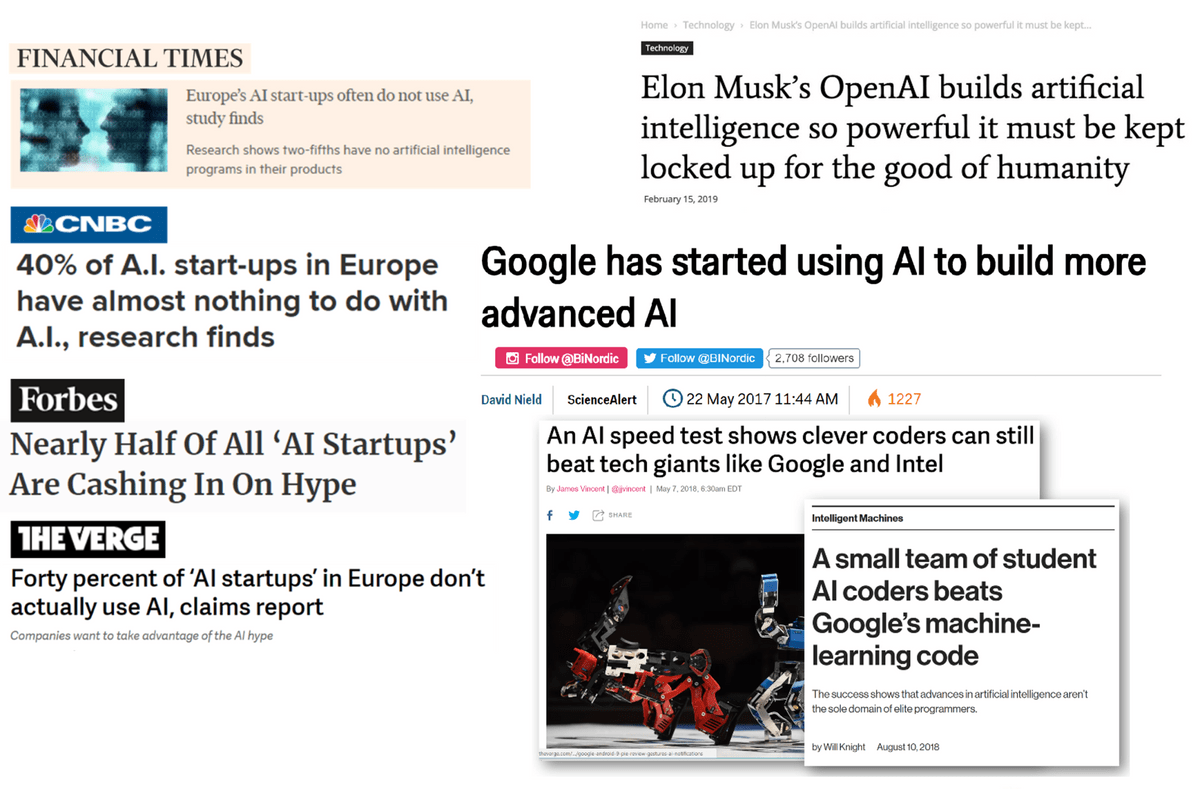
Artificial intelligence is the ability of machines to think like humans. It stems from the idea that given enough data and compute power, machines will be able to think and learn using mathematical simulation of the human brain. Thinking like humans includes concepts like self-learning, reasoning, deciding, correcting, communicating, and—most importantly—increasing in overall intelligence.
According to the Harvard University SITN blog, in an article titled “The History of Artificial Intelligence,” by Rockwell Anyoha, artificial intelligence was first documented by “…Alan Turing, a young British polymath who explored the mathematical possibility of artificial intelligence. Turing suggested that humans use available information as well as reason in order to solve problems and make decisions, so why can’t machines do the same thing? This was the logical framework of his 1950 paper, ‘Computing Machinery and Intelligence,’ in which he discussed how to build intelligent machines and how to test their intelligence.”
AI was conceptualized in the 1950s. It was attempted in the 1960s, 70s, and 80s, but failed to make it to general markets due to insufficient funding and compute power. AI research flourished in the private sector during the 1990s and early 2000s as compute power continued to expand exponentially. The explosion of big data in the early 2010s lead to the resurgence of AI around 2015.
In 2019, as people are bombarded by the term “artificial intelligence,” most of the technology labeled as AI is actually “machine learning.” Machine learning is the data-driven use of advanced algorithms to simulate small parts of human thinking and decision-making processes. Artificial intelligence should, theoretically, be able to increase in intelligence on its own. Most of the smart technology in the market today learns based on the input of more, diverse data and the fine-tuning of advanced mathematical algorithms.
There is still great potential for the use of advanced machine learning to automate decision-making, communicate, and complete tasks normally undertaken by humans. For the purpose of this research, EMA will use both AI and ML to refer to the combined use of both data-driven and self-learning technology.
The Use of AI and ML in Analytics and Data Management
The next major shift in the analytics, business intelligence, and data management markets is coming from the use of AI and ML across the entire information supply chain.
Along with using machine learning to find the next-best offer, companies can now point algorithms at modern data platforms to find links between data sets, automate data preparation, or find breaches in data governance. Technology also exists to automate the heavy lifting of analytic exploration and explanation. All of this is possible by way of a revolution in machine learning, powerful processing, and the availability of enhanced technical, semantic, and operational metadata within data landscapes.
Vendors that excel in the use of AI and ML in their analytics, business intelligence, and data management platforms will create significant differentiation and barriers to entry that will change the face of all industries. Companies that invest in these platforms will also experience an acceleration of insight-driven decisions and gain a competitive advantage.
For a more in depth look at this topic, you can read my full report: “Innovation in the Use of Artificial Intelligence and Machine Learning for Business Intelligence.” Or sign up for my course at TDWI Orlando: AI-Enabled Data Management Tools: Accelerating Analytic Delivery.